Disclaimer
I am not by any means an expert, not even close. I simply love patterns and I am very much a person that reviews the patterns and truths of the past to think about the future. If you read this and find a million reasons it's simply wrong and ill-informed, its because I wrote it on a 2h airplane hop over to Calgary, its very generalized and not researched ....... I would love your thoughts and if anyone out there in the fields of anthropology, evolutionary sciences, genetics, bioinformatics,.... wants to collaborate on a wider discussion I would love to learn from you and your perspectives.
TL/DR - The Evolutionary Parallel in AI
The nature versus nurture framework, traditionally applied to human development, provides a valuable lens for understanding AI training. Just as humans develop through a combination of genetic predispositions (nature) and environmental influences (nurture), AI models evolve through their foundational architecture and the data they are trained on.
- Nature in AI: Refers to the inherent architecture and algorithms—essentially, the 'genetic makeup' of the model.
- Nurture in AI: Corresponds to the training data and experiences the model is exposed to, shaping its learning and capabilities.
Currently, AI models are encountering a 'nurture' bottleneck, as they are running out of diverse, high-quality data. To overcome this, leveraging real-world, contextually rich data from the physical world is essential. This allows AI to develop a deeper, more nuanced understanding, mirroring how humans evolve neurologically through interaction with their environment.
By applying this framework, we aim to create AI that not only mimics human intelligence but evolves alongside it, leveraging the seamless integration of digital and physical realms to drive the next wave of technological advancement.
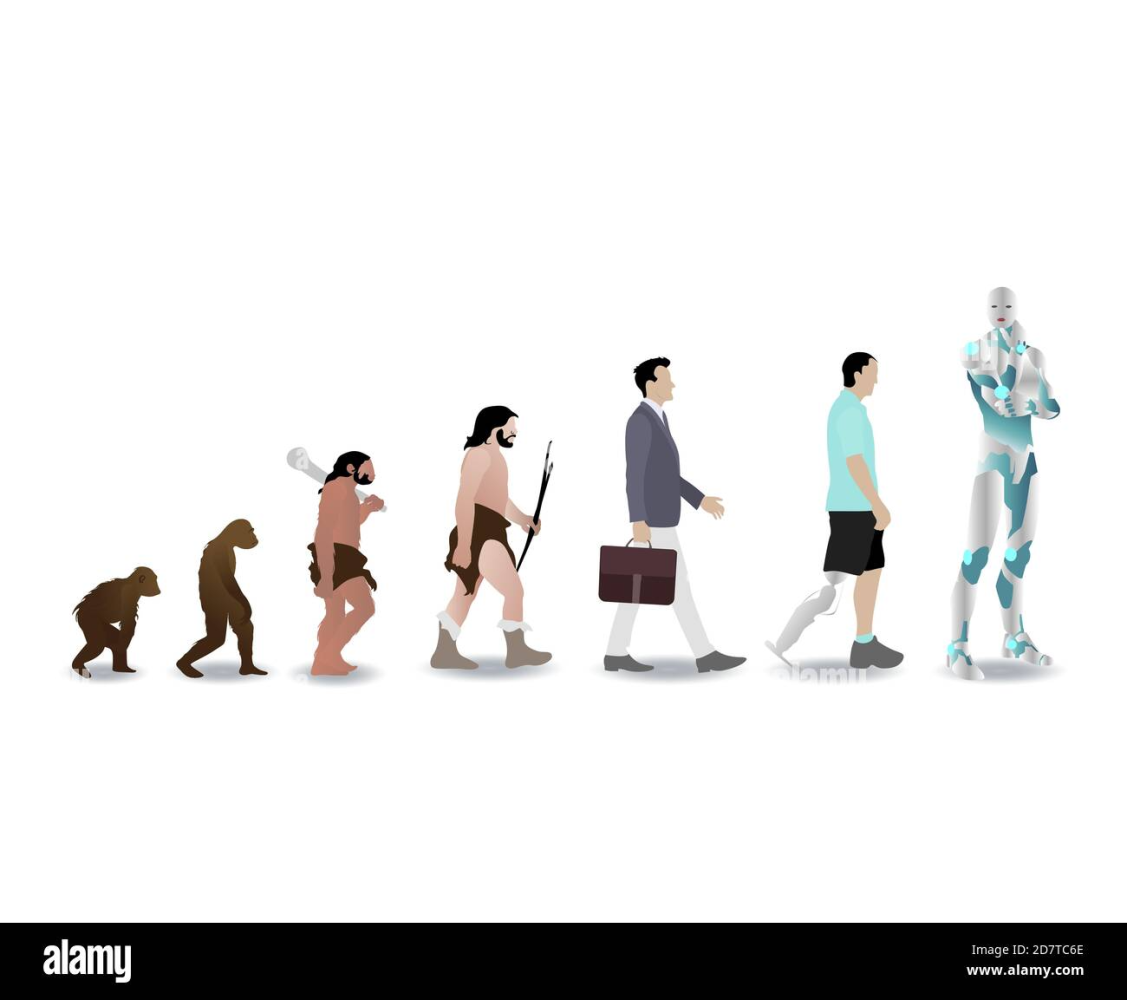
Introduction: The Evolutionary Parallel in AI
The classical nature versus nurture debate in anthropology and psychology provides a valuable lens for examining the evolution of artificial intelligence (AI). Just as humans develop through a combination of genetic predisposition (nature) and environmental exposure (nurture), AI models are shaped by their foundational architecture and the data they are trained on.
Historically, AI development has leaned heavily on the 'nurture' aspect, relying on vast datasets from the digital world to train large language models (LLMs). However, we are approaching a critical inflection point: the availability of high-quality training data is plateauing, limiting the further evolution of AI. This constraint signals the need for a paradigm shift—leveraging real-world, analog data to propel AI into its next frontier.
The Nurture Bottleneck: Running Out of Digital Training Data
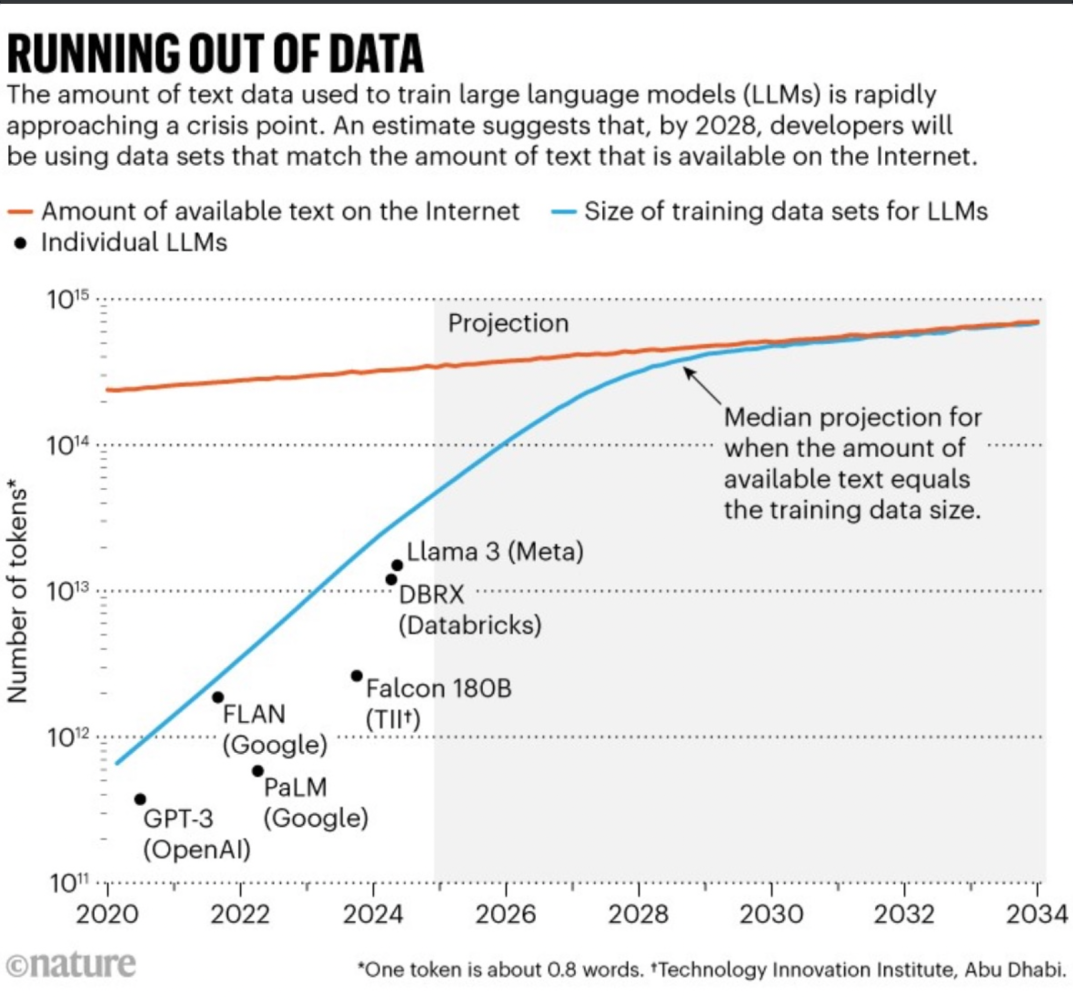
The Limits of Internet-Sourced Data
The current generation of LLMs and its successors has been trained on massive corpora sourced from the internet—books, articles, academic papers, code repositories, and social interactions. However, researchers have raised concerns that we are exhausting this digital wellspring. Studies indicate that most publicly available high-quality text data has already been incorporated into training datasets, leading to diminishing returns on model improvement.
Data Contamination and Diminishing Marginal Returns
Repeated model training cycles on the same datasets lead to data contamination, where models begin reinforcing their own generated outputs, rather than learning from fresh, organic human-generated content. This results in increasing homogeneity and a loss of originality in AI responses.
The Future: Physical Data as the New Frontier
Learning from the Real World: AI’s Next Evolutionary Step
If we map AI’s evolution onto human learning, current LLMs resemble highly intelligent but entirely book-trained individuals, devoid of real-world experience. Humans do not learn in isolation from books or digital content; they learn by interacting with the environment. Similarly, the next phase of AI training will require models to experience and interpret the physical world, integrating sensory inputs beyond text and pixels.
Robotics and Automation as Contextual Data Sources
The most promising approach to overcoming the training plateau is integrating real-world data captured through robotics, sensors, and automation. Unlike static internet-based datasets, physical interactions provide dynamic, context-rich learning. AI models trained on this data can develop:
- Physical intuition: Understanding the physics of objects and interactions, crucial for robotics and automation.
- Situational awareness: Recognizing and adapting to real-world complexities, from environmental changes to human behavior.
- Multimodal cognition: Processing data from multiple sources, including vision, touch, sound, and structured digital inputs.
Conduit's Thesis: Investing and Building at the AI-Physical Nexus
Conduit is positioned at the vanguard of this transition, investing in technologies that bridge the gap between digital AI and real-world interactions. Our investment strategy aligns with the belief that:
- AI evolution will be driven by real-world data sources, requiring the development of new sensors, robotics platforms, and real-time feedback loops.
- Horizontal platform technologies, such as simulation environments, edge computing, and autonomous systems, will be essential enablers for this shift.
- Strategic collaborations with industry, academia, and government dual-use facilities will accelerate the commercialization of AI models that integrate physical-world learning.
Why Founders / Investors Should Care: The New Gold Rush of AI Training Data Is At the Nexus of Physical and Digital
The companies that successfully transition AI from a digitally constrained model to a real-world adaptive intelligence will define the next decade of technological innovation. This shift presents a generational investment opportunity:
- First-mover advantage: Companies at the forefront of integrating real-world training will set the standard for next-gen AI applications in autonomous systems, healthcare, industrial automation, and climate tech.
- Massive market expansion: Current AI applications are limited to digital interactions. Real-world AI unlocks vast new markets in robotics, infrastructure management, and intelligent manufacturing.
- Sustained differentiation: Companies relying solely on static datasets will face stagnation, whereas those leveraging proprietary real-world data sources will maintain a perpetual learning advantage.
🚀 Let's Build